The last fifty years of history have been marked by significant technological developments, especially in the fields of data and digitalization. However, these years have also seen a heated moral debate on the relationship between man and machine, emphasizing the concept of a “crisis.”
Read more about this concept here.
However, if we were to see it with a different perspective, a crisis is an opportunity to bring forth hidden potentials, not only in an individual but also in an entire society.
It is crucial to develop the ability to observe, to learn, to discern, and position various elements related to development correctly. This involves adopting a different mindset towards artificial intelligence, machine learning techniques, and other technologies created and instructed by humans to enhance and simplify certain processes.
Let’s see what happens in the energy sector.
What about renewable energies?
The energy sector is no exception. Digitalization opens new horizons for management systems based on data analysis, increasingly efficient and requiring experts to interpret such sources and create complex algorithms. These algorithms aim to refine predictions for variable and more complex renewable energy sources such as solar, wind, and hydro energy.
The digital world is built on numbers, and the more data collected, the more efficient it becomes. The models created today can predict well in advance which processes and operations will not function optimally or with an efficiency level below expectations. This aids companies in various maintenance operations, recalibration, and restoration interventions. These new technologies are leveraged to optimize and shorten processes, thanks to digitalization and data collection.
The new energy ecosystem
Since the 1970s, with the onset of oil crises, interest in renewable energy sources, particularly photovoltaic solar energy and wind energy, has developed more systematically. Technologies and materials for creating panels, for example, have evolved, becoming more efficient, affordable, and versatile.
As mentioned earlier, the energy ecosystem is evolving and increasing in complexity, requiring stakeholders and those operating within it to adapt rapidly with increasingly advanced tools. These tools include real-time data recording and storage, Internet of Things (IoT), analytics, cloud, and secure distributed infrastructures.
What are smart meters?
Smart meters, such as second-generation meters recently installed in our homes, are one of the necessary tools for the evolutionary transition from traditional measurement systems. These smart meters not only acquire data but also communicate with other devices.
A recent concept: Energy communities, new roles, and the future
Recently, there has been talk of energy communities, the creation of private or public entities that do not centralize but cooperate in creating various multidirectional consumption/production centers. New technologies, tools, such as those mentioned earlier, and new materials help in creating these new production models.
In an energy community, individuals play a dual role—they are consumers and producers of energy simultaneously, known as “prosumers.” By possessing their energy production facility, they consume a portion for their needs and feed the remaining into the grid, exchanging it with other consumers or storing it for later use.
With energy communities, various actors interact together, including individual citizens, industries, businesses, and local authorities, with a common goal: to produce, exchange, and consume energy from renewable sources.
The benefits for an energy community are manifold, including economic aspects, such as the creation of redistributable energy. Producers can reduce costs and consumption, but this is just one aspect to emphasize. There is also a social and educational factor. By stimulating cooperation among people and entities, it raises awareness, by example, of virtuous behaviour and respect for the environment. This approach instils a sense of responsibility for the proper use and management of energy.
Often, blame for mismanagement is externalized. This system triggers important cultural and economic changes; the economy becomes collaborative and participatory, laying the groundwork for the energy future.
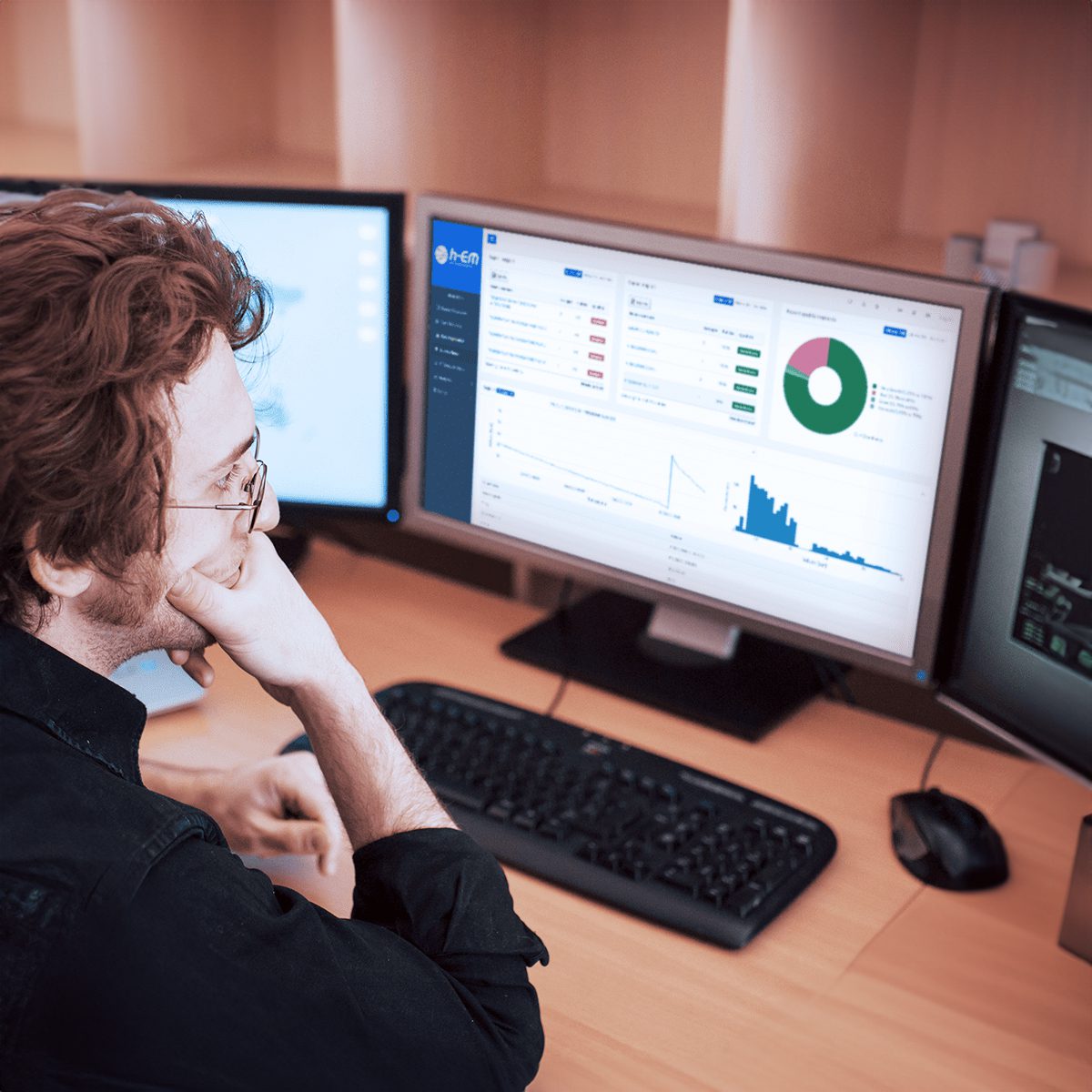
Who is i-EM, what it does, and how it works?
At the heart of the timeless and complex moral dilemma of “man vs. machine,” we find i-EM – Intelligence in Energy Management. Established in 2013 in Livorno as a spin-off of the well-established Flyby, i-EM specializes in developing systems that provide a clear and precise overview of energy plant productivity and forecasting.
i-EM combines the collection of Big Data (satellite data, including weather, environmental, and energy predictions) with machine learning models. These models automatically learn from data, organized by the company’s data scientists, who continually seek innovative algorithms to solve problems and develop specific models for all users.
The Software as a Service (SaaS) developed by the Tuscan company offers detailed and precise analyses for hydropower, wind, solar, network, and electric mobility plants. Operating on more than a thousand plants globally, i-EM is a supplier for major players in the energy industry and smart grids.
As data are the real fuel for i-EM’s machine learning models, the focus is on data quality. This ensures the usability of information, identifies discardable or correctable data through imputation techniques, and prepares them for the actual implementation of the machine learning model.
Scalability is another crucial design pillar. The model’s scalability should allow its implementation on both simple and complex scenarios, accommodating plants of any size. This includes small photovoltaic installations on school roofs to large plants, such as those in the Peruvian desert where i-EM’s solutions have been adopted.
Scalability also applies to processing times. The software built on the machine learning model must be based on an infrastructure that allows modulating calculation times based on process specifications, quantity, and type of data to be processed, and the chosen model for analysing the data.
Once all these aspects are addressed, the model is ready to be “trained.” The training procedure, specific to this approach, usually involves using a historical data archive from which the model learns how to behave based on the data it receives, defining the values of its internal parameters.
The “trained” model, before entering the operational phase, is evaluated based on appropriate data obtained from specific tests. The results of these tests allow adjustments for tuning to optimize accuracy.
Synergistic work
i-EM operates with a synergistic approach between its data scientists and field operators, personnel with specific and long-lasting experience in their sector. This collaboration generates a loop of mutual benefit, enabling the company to develop tools increasingly suitable for decision support and receive essential feedback on these tools. The received feedback allows i-EM to implement improvements to its systems, thanks to the daily and on-field activities of the operators.
The evolution of approach in i-EM
Over time, i-EM has evolved from a descriptive analysis, typical of analog systems, to a diagnostic analysis typical of current digital systems. The aim is to understand why a particular result was produced, providing potential warning signals, and constantly evaluating performance.
Soon, the company anticipates that the analysis will shift more towards predictive analysis. The machine learning models developed will enable predictions of optimal production values and potential issues that may arise in attempting to achieve set goals.
Searching, identifying, and resolving potential issues in advance allows the system to operate more efficiently, minimizing machine downtime and maintenance times. The increasing number of digital management systems being implemented worldwide makes it possible for error prediction applications to constantly improve their predictive capabilities. New solutions can be experimented with and adopted much more rapidly.
i-EM’s first challenge to facilitate the evolution of digital management systems is related to the ability of data analytics algorithms to predict anomalies in electrical system components, especially in energy generation plants. This aims to optimize Operation and Maintenance (O&M) activities and minimize costs.
Through these techniques, it is already possible to predict or detect, with a minimal lead time compared to conventional control systems, potential wear, and degradation of system components. This prevents production losses related to their failure and allows for the planned maintenance of the device, avoiding subsequent extraordinary interventions and ultimately saving thousands of euros.
Practical examples
i-EM develops predictive maintenance models with a particular focus on components of hydropower plants and photovoltaic installations. For example, on a hydropower plant in Campania with four pumping groups and a nominal power of 1 GW, the predictive maintenance model is applied to alternator temperature signals of a generation unit. This solution detected an anomalous condition of overheating, which, through further checks by operators, was confirmed as a potentially severe anomaly on the component. Conventional monitoring systems, not predictive and based on condition monitoring, had not yet identified this issue as the temperatures were still within configured alarm limits.
Early detection of the problem allowed operators to secure the component, avoid group shutdowns, plan device maintenance in a programmed manner, prevent subsequent extraordinary interventions, and ultimately save thousands of euros.
This example represents the perfect combination of the work that humans and machines can do together. Other cases can be listed, such as those related to the electrical grid.
For a specific Italian market area, i-EM worked on predicting peaks in electricity supply demand. By applying nowcasting techniques (short-term forecasts), the goal was to predict anomalous consumption trends not identified by the energy programs of dispatching services. In this case, loads were predicted, and performance was indicated, calculated through the comparison between the model-predicted data and the measured data. This service helps in sizing the electrical system.
With i-EM services, not only loads can be calculated, but also the production of electricity and energy from renewable sources can be predicted. The variability dictated by weather conditions can change rapidly, and without certain tools, it would be challenging to manage.
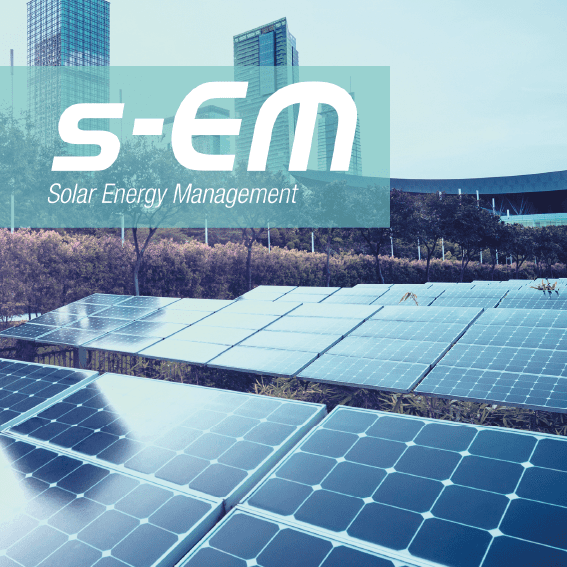
About s-EM
s-EM is a Software as a Service (SaaS) that monitors the real-time status of a solar installation, up to predictive activity. It is a solution that can be integrated into any type of installation, easy to use, intuitive in its operation, and suitable for any type of operator. It is available in standard or advanced diagnostics versions, it can be implemented with various plug-ins to add quality enhancements to your solar installation: solar plant power nowcast and forecast, predictive maintenance, drone data management, solar sensor check, satellite-based plant construction monitoring, self-consumption management.
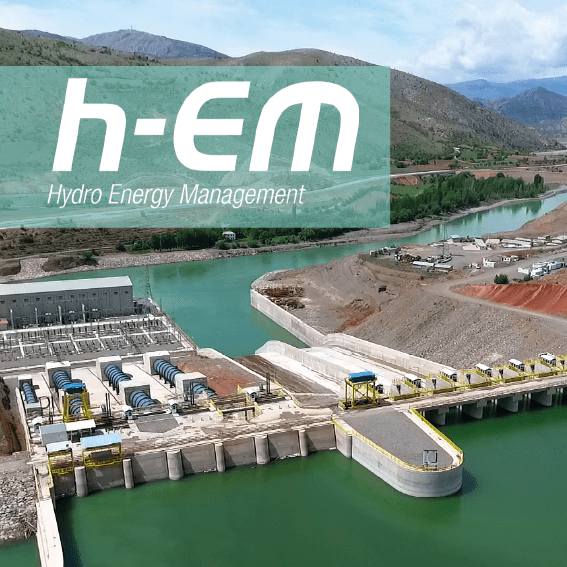
About h-EM
h-EM is a Software as a Service (SaaS) that monitors the real-time status of a solar installation, up to predictive activity. It is a solution that can be integrated into any type of installation, easy to use, intuitive in its operation, and suitable for any type of operator. It is available in standard or advanced diagnostics versions, it can be implemented with various plug-ins to add quality enhancements to your solar installation: solar plant power nowcast and forecast, predictive maintenance, drone data management, solar sensor check, satellite-based plant construction monitoring, self-consumption management.
In conclusion
Balance is the answer to the complex moral and timeless dilemma: human or machine. Consciously embracing technologies, recognizing their benefits, and refining various models emerge as the key challenge for the contemporary human being.
Digitalization, with its vast data collection, has given rise to extraordinary analysis and decision support tools. These tools not only improve individual life but also transform entire communities, redefining roles and making humans active protagonists in energy production. This entails a different quality of responsibility, urging us to become proactive actors in shaping the world through our daily choices. In this way, modern humans can leverage technologies for collective good, becoming architects of a more aware and responsible future.
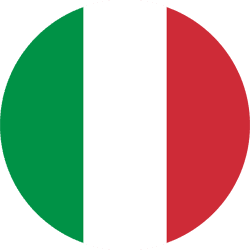
The entire article in Italian language published in the Nuova Atlantide magazine, click here to read.
Keywords
Analytics, Data Science, Data Analytics, Data Acquisition, Data Exploration, Solar Forecast, Solar Nowcast, Solar Power Prediction, Grid Management, Smartgrid, Satellite, Space